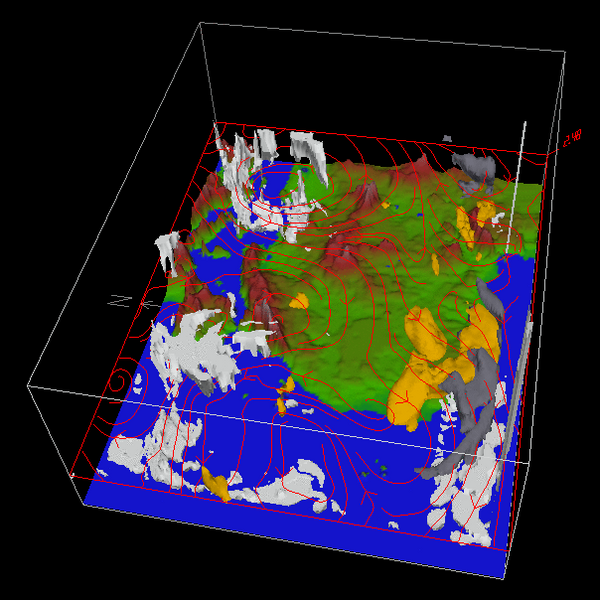
Model simulation of clouds (white), wind fields (red lines), fire aerosol (grey) and mineral dust (yellow) over western Africa and the Atlantic. Source: Bernd Heinold/TROPOS
Model simulation of clouds (white), wind fields (red lines), fire aerosol (grey) and mineral dust (yellow) over western Africa and the Atlantic. Source: Bernd Heinold/TROPOS
Overview
COSMO-MUSCAT is a modern mesoscale chemistry transport model for process studies and air quality applications (Wolke et al., 2012). The online-coupled system consists of the regional weather model COSMO (COnsortium for Small scale MOdelling) and the chemistry transport model MUSCAT (MUltiScale Chemistry Aerosol Transport). COSMO, which until 2020 was the operational forecast model of the German Weather Service (DWD), solves the atmospheric equations on the basis of a terrain-following grid (Schättler et al., 2018; Baldauf et al., 2011). Driven by the meteorological model, MUSCAT deals with atmospheric transport and chemical transformations for various gas-phase species and aerosol particle populations. Its core is based on mass balances, which are described by a system of time-dependent, three-dimensional advection-diffusion-reaction equations.
MUSCAT contains current emission parameterizations for natural aerosols, such as desert dust (Heinold et al., 2011; Schepanski et al., 2017) and aerosol from marine sources (Barthel et al., 2019), as well as a sophisticated scheme for biogenic emissions (Luttkus et al., 2021). The formation of secondary organic aerosol (SOA) and complex multiphase chemical processes are considered in great detail (Schrödner et al., 2014; Luttkus et al., 2021). Aerosol particles can interact with clouds and radiation in the meteorological model and act on atmospheric dynamics. The anthropogenic emissions of primary particles and precursors of secondary aerosols are specified from current cadastres. The transport processes include advection, turbulent diffusion, sedimentation, dry and wet deposition. The driving wind fields as well as the vertical diffusion coefficients and boundary layer resistances are provided online by COSMO (Raschendorfer, 2001). In addition, the model is powered by Double Canyon Effect Parametrization (DCEP; Schubert et al., 2012) to take into account the effects of urban morphology on air flow and radiation in urban simulations.
COSMO-MUSCAT is regularly used for air quality applications (e.B. Stern et al., 2007; Hinneburg et al., 2009; Renner und Wolke, 2010; Wolke et al., 2012; Luttkus et al., 2021). In addition, the model is used in various scientific studies in which, for example, the direct and semi-direct radiation effects of absorbing aerosols are investigated (e.B. Heinold et al., 2011; Meier et al., 2012; Banks et al., 2018) or the influence of aerosol particles on the budget of cloud condensation (Dipu et al., 2017) and ice nuclei (Weger et al., 2019). In 2019, COSMO-MUSCAT was used for the first time for an operational air quality forecast for the city of Leipzig and eastern Central Germany to accompany a field campaign with citizen scientists (Tõnisson et al., 2021).
Several model comparison studies confirm the good performance of the model system, e.g. the international model evaluation initiative AQMEII (Solazzo et al., 2012 a,b; In et al. 2014 a,b).
Scheme of the chemistry transport model COSMO-MUSCAT.
Maximum hourly NO2 load over Central Germany from the quasi-operational forecast with COSMO-MUSCAT for 24.09.2019. Screenshot of the interactive web map provided as part of the citizen science project WTimpact.
Atmospheric aerosols and trace gases
Atmospheric aerosols and trace gases
Multiphase chemistry. Gas-phase processes, including the formation of photooxidants and the transport and conversion of particles, are taken into account. Chemical reaction mechanisms are imported from ASCII files. All information required for the calculation of the chemical terms and the associated Jacobi matrix is generated from such a mechanism file. Changes in the chemical mechanism are therefore easily and comprehensively possible. Several gas-phase mechanisms, e.g. RACM-MIM2-ext (Stockwell et al., 1997; Karl et al., 2006; Luttkus et al., 2021) with more than 90 species and over 200 reactions, have been successfully used in 3D-Fall studies. Recently, the gas-phase chemistry can also be described by the current MOZART4/JAM-002 mechanism with over 200 species and over 500 reactions (Schultz et al., 2018). All gas-phase mechanisms deal with the degradation of biogenic and anthropogenic volatile compounds (VOCs) with varying degrees of complexity. An important issue in the chemical conversion of VOCs is their impact on ozone concentration (O3), which is to be taken into account in MUSCAT.
The formation of secondary organic aerosol (SOA) is described by the aerosol module SORGAM (Schell, et al., 2001), which has been extended by additional production pathways, including the reactions of monoterpenes with NO3 (Griffin et al., 1999), isoprene (Kroll et al. , 2006; Ng et al., 2008), sesquiterpene (Hoffmann et al., 1997; Karl et al., 2009b) as well as the formation of highly oxygenated organic molecules (HOMs) from all considered BVOCs (monoterpenes: Berndt et al., 2016a; Jokinen et al., 2015; sesquiterpene: Richters et al., 2016; Isoprene: Berndt et al., 2016b). Tests showed that these model extensions significantly improve the correspondence of simulated and measured concentrations of organic matter (Luttkus et al., 2021).
Secondary inorganic aerosol formation is calculated from reactions between ammonia and sulfuric or nitric acid formed from the gas-phase precursors SO2 and NOX (Hinneburg et al., 2009). The particle/gas partitioning applied depends on the temperature and humidity. As in ISORROPIA (Nenes et al., 1998), the equilibrium is shifted towards the gas phase in dry and warm conditions. The realization is comparable to Galperin and Sofiev (1998) and uses the equilibrium approach of Mozurkewich (1993). N2O5 hydrolysis, which is an important source of aerosol nitrate in the troposphere, is parameterized according to Chen et al. (2018).
The photolysis rates used in MUSCAT chemistry are given in the mechanism file as rates for cloudless sky in the reaction-specific wavelength range according to MCM regulations and are dependent on the solar zenith angle (Saunders et al. 2003). Shading factors for the reduction of photolysis rates due to clouds are calculated from COSMO diagnostics of vertically resolved cloud cover and high cloud cover for layers above the MUSCAT domain (Eppel et al., 1995). The modelled aerosol and ozone concentration is currently not taken into account.
For a detailed description of the chemistry of the aqueous phase, a reduced CAPRAM scheme was integrated into the model system (Schrödner et al., 2014; 2018).
Aerosol dynamics. There are two approaches to describing the different aerosol populations in MUSCAT. On the one hand, there is a hybrid bulk bin scheme that is used as the standard configuration for air quality simulations. It includes 25 prognostic particle tracers, including primary PM2.5 and PM10,primary organic carbon (POC), black carbon (BC), sulfate aerosol, nitrate and ammonium, secondary organic aerosol (SOA), as well as six bins for sea salt and primary marine organic particles (diameter range: 0.01-10 μm) and five desert dust bins (0.2-48 μm). The different aerosolarten are considered externally mixed. In addition, the modal aerosol scheme M7 (Vignati et al., 2004), extended by the treatment of nitrate and ammonium, can be used to describe the particle size distribution and dynamic aerosol processes. In this approach, the entire particle population is aggregated from seven lognormal modes with different compositions depending on the size and solubility of the aerosol particles. The size distribution develops due to extern dynamic aerosol processes such as particle transport by convection and diffusion, deposition and sedimentation, as well as due to internal processes such as condensation and coagulation.
Anthropogenic emissions. The temporally resolved anthropogenic emissions are treated as point, area and line sources. The temporal evolution of anthropogenic emissions is considered in terms of intensity on the basis of time profiles, distinguishing between the different emission sectors. The different types of sources (e.g. energy conversion, industrial combustion, road transport, agriculture) can be specified either in the form of SNAP codes (Selected Nomenclature of Air Pollution) or using the GNFR classification (Gridded Nomenclature For Reporting) for the source sectors. The emission files of gases and aerosols within MUSCAT are generated by preprocessors. Within Europe, the regional cadastre of anthropogenic aerosol and trace gas emissions CAMS-REG (Kuenen et al., 2014; Granier et al., 2019; eccad3.sedoo.fr) of the Copernicus Atmosphere Monitoring Service (CAMS), while outside Europe air pollutant emissions are based on the EDGARv5 dataset (Crippa et al., 2018). In addition, emissions from biomass combustion are compared with daily data from the Global Fire Assimilation System (GFAS; Kaiser et al., 2012). For applications in Germany, point and detailed area (550 m native solution) and road emissions from the GRETA database of the Federal Environment Agency (UBA; Scheider et al., 2016). The temporal emission profiles for the most important air pollutants and greenhouse gases are consistently based on the CAMS-REG-TEMPO dataset for Europe and Germany.
Biogenic emissions. Agricultural land can be an important source of nitrogen oxides caused by microbiological processes. Soil NO emissions are parameterized using empirical relationships that depend on land use type, seasonal phenology, and soil temperature. Functional dependence follows Williams et al. (1992) and Stohl et al. (1996).
For BVOC emissions, the model is used by Steinbrecher et al. (2009), which takes into account the dependence on meteorological parameters (photosynthesis radiation, temperature) and land use: (1) area of a given land use category, (2) associated biomass density (BD) and (3) standard emission potential (SEP at 30°C and photosynthesis radiation of 1000 mol m 2 s 1) for various BVOC as well as seasonality. The values for the agricultural BD have been updated on the basis of Oderbolz et al. (2013). The BVOC emissions considered include isoprene, 17 monoterpenes (e.B. alpha- and beta-pinene, limonene), sesquiterepenes and oxygenated BVOCs (e.B alcohols, aldehydes). Each BVOC species has a specific reaction rate coefficient for the reaction with ozone, OH and NO3 radicals and can thus influence the atmospheric oxidation potential. The strength and composition of BVOC emissions varies significantly between land use species/tree species due to the associated BD and their BVOC-specific SEP. The standard setup uses a dataset of 10 land use classes: water, mudflats, sand, mixed land use (mainly agriculture), meadows, heath, bushes, mixed forest, coniferous forest, and urban area. In addition, a new, more detailed land use data set has been introduced, covering 138 land use classes with 116 tree species and 11 agricultural land use types (Köble and Seufert, 2001). A sensitivity study using the two setups showed that a too general model representation of land use with only a simple categorization into mixed land use, coniferous and mixed forests regionally leads to 50% overestimated BVOC emissions (isoprene, mono- and sesquiterpenes), resulting in altered oxidant concentrations (NOx 2.5%, ozone +2.5%, OH 50%, NO3 radical -70%) and an overestimation of SOA by up to 60% (Luttkus et al., 2021). OzonEval uses CORINE land cover data, which include similar agricultural land use classes to the Köble and Seufert et al. dataset (2001), but generalized forest categories.
Wind-induced aerosol emissions. As the two main components of the natural aerosol, MUSCAT calculates desert dust and marine aerosol online. The calculation of desert dust mobilization uses the current wind fields and hydrological conditions from the COSMO model and takes into account soil texture and soil size distribution according to Tegen et al. (2002) as well as preferential source regions (Heinold et al., 2011; Schepanski et al., 2017). The sea salt emissions are calculated with a parameterization according to Long et al. (2011) taking into account the dependence on the sea surface temperature according to Sofiev et al. (2011). In conjunction with sea salt, the emission of primary marine aerosol is based on the distribution of marine chlorophyll-a (Barthel et al., 2019).
Dry and wet deposition. Dry deposition is modeled using the resistance approach used by Seinfeld and Pandis (2006), which takes into account atmospheric turbulence, kinetic viscosity and the deposition of particles by gravity. The calculation includes the quasi-laminar, the turbulent roughness and the turbulent Prandtl layer (aerodynamic) resistance, each online from COSMO (Raschedorfer, 2001; Baldauf et al., 2011) and thus directly depend on the boundary layer development and the land surface properties of the meteorological driver model. In addition, the surface resistance is parameterized as a function of land use (Schlünzen & Pahl 1991; Schlünzen et al. 2012). Aerosol particles and trace gases are also removed from the atmosphere by wet deposition, distinguishing between in-cloud and below-cloud scavenging. Both processes are parameterized in the form of size-dependent collection efficiencies for particles and corresponding absorption coefficients for gases (Simpson et al., 2012).
Numerical
Multiblock grid structure. In MUSCAT, a static horizontal grid staggering (Wolke and Knoth, 2000; Knoth and Wolke, 1998b). The meteorological model COSMO uses a rotated spherical grid with a hybrid vertical coordinate. To distribute the horizontal grid across all processors, it is broken down into rectangular partitions with as many grid cells as possible. The MUSCAT grid is based on that of the COSMO, but is placed in so-called blocks, which can have different horizontal resolutions. This multi-block technology is used to reduce computing costs in less interesting border regions and to concentrate on certain regions of interest, such as power stations and urban areas, with a finer resolution. Spatial discretization is performed by a finite volume scheme on a staggered lattice. Such schemes are known to be mass conservative due to the direct discretization of the integral measures of conservation theorems. For the approximation of the surface integrals, point values of the mixing and their first discharge on the cell surfaces are required. To approximate the mixing ratio at the surface, both a first-order upwind method and a third-order upwind-biased method with additional limitation are used (Hundsdorfer et al., 1995). This method must be applied to non-equidistant stencils occurring at the intersection of blocks with different resolutions (Knoth and Wolke, 1998b). The partitions in MUSCAT are formed by assigning blocks to processors. This assignment is determined using the grid partitioning library ParMETIS (Karypis et al., 2003). It minimizes the length of the partition boundaries ("edge cut") while balancing the number of grid cells of each processor. The more blocks are used for decomposition, the finer the number of cells can be balanced.
Time integration scheme. MUSCAT uses an implicit-explicit (IMEX) time integration scheme (Knoth and Wolke, 1998b; Wolke and Knoth, 2000) to efficiently combine the slow process of horizontal advection and the fast processes of vertical exchange and gas-phase chemistry. While the slow processes are integrated by explicit Runge-Kutta methods, any suitable solver can be used for the fast processes. Discontinuities in the solution process as with conventional operator splitting are avoided with this approach. As part of the implicit integration in the chemistry transport code MUSCAT, rigid chemistry and all vertical transport processes (turbulent diffusion, advection, deposition) are integrated coupled with the BDF method of the second order. We use a modification of the code LSODE (Hindmarsh, 1983) with a special linear system solver and an adapted restart method (Knoth and Wolke, 1998a). Error control can result in multiple implicit time steps per explicit step. In addition, different implicit step sizes can be created in different blocks. The size of the "large" explicit time step depends on the CFL number. By selecting different advection steps in different model regions, a significant reduction in computing costs can be achieved, especially in cases with few large point sources (Schlegel et al., 2012a, b).
Online coupling meteorology--chemistry. In the COSMO-MUSCAT model system, two different coupling strategies can alternatively be used (Lieber and Wolke, 2008). In the parallel approach, both model codes run largely independently of each other on their own disjoint set of processors. The number of processors for meteorology and chemical transport must be determined during model initialization. Since MUSCAT uses adaptive time step control, the total load fluctuates during runtime, especially in scenarios with highly dynamic behavior of the simulated chemical processes. These fluctuations led to idle times of the processor at the synchronization points of the two models. Fluctuations in the total MUSCAT workload resulted in idle times of the processors at the synchronization points of the two models. In order to achieve higher efficiency, an alternative coupling scheme based on the "sequential" approach was implemented (Lieber und Wolke, 2008). The advantages are increased performance and simplified model design, as no processor partitioning (determination of processors for COSMO and MUSCAT) has to be defined a priori. The fact that this crucial decision does not have to be made eliminates a potential source of inefficiency. The codes are synchronized only for data exchange between COSMO and MUSCAT. This only happens in each explicit time step (pair time step). Since this step is chosen as a fraction of the CFL number, its length varies over the prediction time. Fig. 4 shows the coupling scheme.
Parallelization and dynamic load balancing. The code is parallelized by distributing the blocks (rectangular subsets of the grid) to the available processors using MPI. Static splitting can lead to load imbalances because each block has its own time step size defined by the implicit time integrator. Therefore, a dynamic load balancing system was developed that periodically redistributes the blocks. The ParMETIS graph partitioning library (Karypis et al., 2003) is used to identify improved partitioning from the workload of the blocks and their neighborhood.
Nesting chain for air quality modelling with COSMO-MUSCAT for the city of Leipzig.
Default-Configuration
Air quality simulations with COSMO-MUSCAT are typically performed in a nested approach. Starting from an outer domain, the horizontal resolution will be gradually increased from 14 km over Europe to 2 - 4 km above Germany and to about 500 m for the innermost domain, which covers the urban area and the surroundings of a city of interest. In the vertical, the model is used with a height-based, terrain-following coordinate with increasing layer thickness with height. For the calculation areas with a grid spacing of 14 km, 2 - 4 km and 500 m respectively, a setup with 40, 65 and 60 layers is used, with 11, 20 andd 23 vertical layers within the lowest 1000 m. The lowest layer extends up to 20 m above the ground and 7 m for the 500 m area. The top of the model in COSMO is located about 22 km above ground and 15 km for the 500 m area, while the vertical coordinate of MUSCAT is limited to 8 km altitude. The outermost domain, which provides the background concentrations of aerosols and chemical substances over Germany, is driven by the ICON-EU reanalysis of the German Weather Service (DWD). The CAMS model prediction provides the input for the aerosol and gas boundary conditions of the coarse domain. The meteorological initial and marginal data for the area over Germany with a grid spacing of 2.2 km come from the DWD reanalysis COSMO-D2 or ICON-D2. For the following two domains with a grid width of 1.1 km and 550 m respectively, the boundary conditions of the coarser model run are used. For the European domain, anthropogenic air pollutant emissions based on the CAMS-REG cadastre and land use are specified by the EU-JRC data based on CORINE 2000 with detailed tree species information from Köble and Seufert (2001), while for Germany the GRETA emissions and the ESA GlobCover 2009 database are used. The model runs are reinitialized every 48 to 72 hours to keep the meteorology close to the real weather conditions. After 24 hours of spin-up time for COSMO, MUSCAT is coupled to calculate the chemical and transport processes. The first cycle of a simulation period begins with fixed initial concentrations or CAMS air pollution fields; the following cycles are initialized with the trace gas and aerosol fields of the previous run to ensure continuous simulation.
References
Baldauf, M., Seifert, A., Förstner, J., Majewski, D., Raschendorfer, M. & Reinhardt, T. (2011). Operational convective-scale numerical weather prediction with the COSMO model: description and sensitivities. Mon. Weather Rev. doi:10.1175/MWR-D-10-05013.1.
Banks, J. R., Schepanski, K., Heinold, B., Hünerbein, A., and Brindley, H. E.: The influence of dust optical properties on the colour of simulated MSG-SEVIRI Desert Dust infrared imagery, Atmos. Chem. Phys., 18, 9681–9703, doi.org/10.5194/acp-18-9681-2018, 2018.
Barthel, S., Tegen, I. & Wolke, R. (2019). Do new sea spray aerosol source functions improve the results of a regional aerosol model? Atmos. Environ., 198, 265-278. doi.org/10.1016/j.atmosenv.2018.10.016.
Berndt, T., Herrmann, H., Sipilä, M., & Kulmala, M. (2016). Highly Oxidized Second-Generation Products from the Gas-Phase Reaction of OH Radicals with Isoprene. J. Phys. Chem. A, 120, 10150–10159. doi.org/10.1021/acs.jpca.6b10987.
Berndt, T., Richters, S., Jokinen, T., Hyttinen, N., Kurtén, T., Otkjær, R. V., et al. (2016). Hydroxyl radical-induced formation of highly oxidized organic compounds. Nat. Comm., 7(13677), 1–8. doi.org/10.1038/ncomms13677.
Chen, Y., Wolke, R., Ran, L., Birmili, W., Spindler, G., Schröder, W., Su, H., Cheng, Y., Tegen, I., & Wiedensohler, A. (2018). A parameterization of the heterogeneous hydrolysis of N2O5for mass-based aerosol models: improvement of particulate nitrate prediction. Atmos. Chem. Phys., 18, 673–689. doi.org/10.5194/acp-18-673-2018, 2018.
Crippa, M., Guizzardi, D., Muntean, M., Schaaf, E., Dentener, F., van Aardenne, J. A., Monni, S., Doering, U., Olivier, J. G. J., Pagliari, V., & Janssens-Maenhout, G. (2018). Gridded emissions of air pollutants for the period 1970–2012 within EDGAR v4.3.2. Earth Syst. Sci. Data, 10, 1987–2013. doi.org/10.5194/essd-10-1987-2018.
Dipu, S., Quaas, J., Wolke, R., Stoll, J., Mühlbauer, A., Sourdeval, O., Salzmann, M., Heinold, B. & Tegen, I. (2017). Implementation of aerosol–cloud interactions in the regional atmosphere–aerosol model COSMO-MUSCAT(5.0) and evaluation using satellite data.Geosci. Model Dev., 10, 2231-2246. doi.org/10.5194/gmd-10-2231-2017.
Eppel, D. P., Kapitza, H., Claussen, M., Jacob, D., Koch, W., Levkov, L., Mengelkamp, H.-T. & Werrmann, N. (1995). The Non-Hydrostatic Mesoscale Model GESIMA. Part II: Parameterizations and Applications, Beitr. Phys. Atmosph., 68, 15-41.
Galperin, M. V. & Sofiev, M. A. (1998). The long-range transport of ammonia and ammonium in the northern hemisphere. Atmos. Environ. 32, 373-380.
Gatzsche, K., Iinuma, Y., Tilgner, A., Mutzel, A., Berndt, T. & Wolke, R. (2017). Kinetic modeling studies of SOA formation from α-pinene ozonolysis. Atmos. Chem. Phys., 17, 13187–13211. doi.org/10.5194/acp-17-13187-2017.
Granier, C., S. Darras, H. Denier van der Gon, J. Doubalova, N. Elguindi, B. Galle, M. Gauss, M. Guevara, J.-P. Jalkanen, J. Kuenen, C. Liousse, B. Quack, D. Simpson & K. Sindelarova (2019). The Copernicus Atmosphere Monitoring Service global and regional emissions (April 2019 version). Report April 2019 version. doi:10.24380/d0bn-kx16.
Griffin, R. J., Cocker III, D. R., Flagan, R. C., & Seinfeld, J. H. (1999). Organic aerosol formation from the oxidation of biogenic hydrocarbons. J. Geophys. Res.: Atmos., 104(D3), 3555–3567. doi.org/10.1029/1998JD100049.
Heinold, B., Tegen, I., Schepanski, K., Tesche, M., Esselborn, M., Freudenthaler, V., Gross S., Kandler K., Knippertz, P., Müller, D., Schladitz, A., Toledano, C., Weinzierl, B., Ansmann, A., Althausen, D., Müller, T., Petzold, A. & Wiedensohler, A. (2011). Regional modelling of Saharan dust and biomass-burning smoke Part 1: Model description and evaluation. Tellus B, 63(4), 781-799. doi:10.1111/j.1600-0889.2011.00570.x.
Hindmarsh, A. C. (1983). ODEPACK: A systematized collection of ODE solvers. In: R. S. Stepleman, Ed., Scientific Computing, pages 55–74.
Hinneburg, D., Renner, E. & Wolke, R. (2009). Formation of secondary inorganic aerosols by power plant emissions exhausted through cooling towers in Saxony. Environ. Sci. Pollut. Res., 16, 25-35.
Hoffmann, T., Odum, J. R., Bowman, F., Collins, D., Klockow, D., Flagan, R. C., & Seinfeld, J. H. (1997). Formation of Organic Aerosols from the Oxidation of Biogenic Hydrocarbons. J. Atmos. Chem., 26(2), 189–222. doi.org/10.1023/A:1005734301837.
Hundsdorfer, W., Koren, B., van Loon, M. & Verwer, J. G. (1995). A positive finite-difference advection scheme. J. Comput. Phys. 117, 35–46.
Im, U., Bianconi, R., Solazzo, E., Kioutsioukis, I., Badia, A. et al. (2014a). Evaluation of operational online-coupled regional air quality models over Europe and North America in the context of AQMEII phase 2. Part I: Ozone. Atmos. Environ., 115, 404-420. doi.org/10.1016/j.atmosenv.2014.09.042.
Im, U., Bianconi, R., Solazzo, E., Kioutsioukis, I., Badia, A., et al. (2014b). Evaluation of operational online-coupled regional air quality models over Europe and North America in the context of AQMEII phase 2. Part II: Particulate matter. Atmos. Environ., 115, 420-441, doi:10.1016/j.atmosenv.2014.08.072.
Jokinen, T., Berndt, T., Makkonen, R., Kerminen, V.-M., Junninen, H., Paasonen, P., et al. (2015). Production of extremely low volatile organic compounds from biogenic emissions: Measured yields and atmospheric implications. PNAS, 112(23), 7123–7128. doi.org/10.1073/pnas.1423977112.
Kaiser, J. W., Heil, A., Andreae, M. O., Benedetti, A., Chubarova, N., Jones, L., Morcrette, J.-J., Razinger, M., Schultz, M. G., Suttie, M. & van der Werf, G. R. (2012). Biomass burning emissions estimated with a global fire assimilation system based on observed fire radiative power, Biogeosciences, 9, 527–554. doi.org/10.5194/bg-9-527-2012.
Karl, M., Dorn, H.-P., Holland, F., Koppmann, R., Poppe, D., Rupp, L., Schaub, A., Wahner, A. (2006). Product study of the reaction of OH radicals with isoprene in the atmosphere simulation chamber, SAPHIR. J. Atmos. Chem., 55, 167-187.
Karl, M., Guenther, A., Koble, R., Leip, A., & Seufert, G. (2009a). A new European plant-specific emission inventory of biogenic volatile organic compounds for use in atmospheric transport models. Biogeoscience, 6, 1059–1087. doi.org/10.5194/bg-6-1059-2009.
Karl, M., Tsigaridis, K., Vignati, E., & Dentener, F. (2009b). Formation of secondary organic aerosol from isoprene oxidation over Europe. Atmos. Chem. Phys., 9(18), 7003–7030. doi.org/10.5194/acp-9-7003-2009.
Karypis, G., Schloegel, K. & Kumar, V. (2003). ParMETIS: Parallel graph partitioning and sparse matrix ordering library (Version 3.1). University of Minnesota.
Kioutsioukis, I., Im, U., Solazzo, E., Bianconi, R., Badia, A., et al. (2016). Insights into the deterministic skill of air quality ensembles from the analysis of AQMEII data, Atmos. Chem. Phys., 16, 15629–15652. doi.org/10.5194/acp-16-15629-2016.
Knoth, O. & R. Wolke (1998a). An explicit-implicit numerical approach for atmospheric chemistry-transport modelling. Atmos. Environ. 32, 1785-1797.
Knoth, O. & R. Wolke (1998b). Implicit-explicit Runge-Kutta methods for computing atmospheric reactive flows, Appl. Numer. Math. 28, 327–341.
Köble, R., & Seufert, G. (2001). Novel Maps for Forest Tree Species in Europe. Paper presented at Proceedings of the 8th European Symposium on the Physico-Chemical Behaviour of Air Pollutants: "A Changing Atmosphere!", Torino, Italy.
Kroll, J. H., Ng, N. L., Murphy, S. M., Flagan, R. C., & Seinfeld, J. H. (2006). Secondary Organic Aerosol Formation from Isoprene Photooxidation. Environ. Sci. Technol., 40(6), 1869–1877. doi.org/10.1021/es0524301.
Kuenen, J. J. P., Visschedijk, A. J. H., Jozwicka, M. & Denier van der Gon, H. A. C. (2014). TNO-MACC_II emission inventory; a multi-year (2003–2009) consistent high-resolution European emission inventory for air quality modelling. Atmos. Chem. Phys., 14, 10963–10976. doi.org/10.5194/acp-14-10963-2014.
Lieber, M. & Wolke, R. (2008). Optimizing the coupling in parallel air quality model systems. Environ. Model. Softw., 23, 235-243.
Long, M. S., Keene, W. C., Kieber, D. J., Erickson, D. J. & Maring, H. (2011). A sea-state based source function for size- and composition-resolved marine aerosol production. Atmos. Chem. Phys., 11, 1203–1216. doi:10.5194/acp-11-1203-2011.
Luttkus, M. L., Hoffmann, E. H., Poulain, L., Tilgner, A. & Wolke, R. (2021). The effect of land use classification on the gas-phase and particle composition of the troposphere: tree species vs. forest information. J. Geophys. Res.: Atmos., submitted.
Meier, J., Tegen, I., Heinold, B. & Wolke, R. (2012). Direct and semi-direct radiative effects of absorbing aerosols in Europe: Results from a regional model. Geophys. Res. Lett., 39, L09802. doi:10.1029/2012GL050994.
Mozurkewich, M. (1993). The dissociation constant of ammonium nitrate and its dependence on temperature, relative humidity and particle size. Atmos. Environ., 27 A, 261-270.
Ng, N. L., Kwan, A. J., Surratt, J. D., Chan, A. W. H., Chhabra, P. S., Sorooshian, A., et al. (2008). Secondary organic aerosol (SOA) formation from reaction of isoprene with nitrate radicals (NO3). Atmos. Chem. Phys., 8(14), 4117–4149. https://doi.org/10.5194/acp-8-4117-2008.
Oderbolz, D. C., Aksoyoglu, S., Keller, J., Barmpadimos, I., Steinbrecher, R., Skjøth, C. A., et al. (2013). A comprehensive emission inventory of biogenic volatile organic compounds in Europe: improved seasonality and land-cover. Atmospheric Chemistry and Physics, 13(4), 1689–1712. doi.org/10.5194/acp-13-1689-2013.
Raschendorfer, M. (2001). The new turbulence parameterization of LM. COSMO Newsletter, 1, 89–97.
Renner, E. & Wolke, R., (2010). Modelling the formation and atmospheric transport of secondary inorganic aerosols with special attention to regions with high ammonia emissions. Atmo. Environ., 44, 1904-1912.
Richters, S., Herrmann, H., & Berndt, T. (2016). Highly Oxidized RO2Radicals and Consecutive Products from the Ozonolysis of Three Sesquiterpenes. Environ. Sci. Technol., 50(5), 2354–2362. doi.org/10.1021/acs.est.5b05321.
Saunders, S. M., Jenkin, M. E., Derwent, R. G. & Pilling, M. J. (2003). Protocol fort he development oft he Master Chemical Mechanism, MCM v3 (Part A): tropospheric degradation of non-aromatic volatile organic compounds. Atmos. Chem. Phys., 3, 161-180. doi.org/10.5194/acp-3-161-2003.
Schättler, U., Doms, G. &Schraff, C. (2018). A Description of the Nonhydrostatic Regional COSMO-Model. Deutscher Wetterdienst, Offenbach. www.cosmo-model.org.
Schell, B., Ackermann, I. J., Hass, H., Binkowski, F. S., & Ebel, A. (2001). Modeling the formation of secondary organic aerosol within a comprehensive air quality model system. J. Geophys. Res.: Atmos.,106(D22), 28275–28293. doi.org/10.1029/2001JD000384.
Schepanski, K., Heinold, B. & Tegen, I. (2017). Harmattan, Saharan heat low, and West African monsoon circulation: modulations on the Saharan dust outflow towards the North Atlantic. Atmos. Chem. Phys., 17, 10223-10243. doi.org/10.5194/acp-17-10223-2017.
Schlegel, M., Knoth, O., Arnold, M. & Wolke, R. (2012a). Implementation of multirate time integration methods for air pollution modelling. Geosci. Model Dev., 5, 1395–1405. doi.org/10.5194/gmd-5-1395-2012.
Schlegel, M., O. Knoth, M. Arnold, & R. Wolke (2012b). Numerical solution of multiscale problems in atmospheric modeling. Appl. Numer. Math., 62(10), 1531-1543. doi:10.1016/j.apnum.2012.06.023.
Schlünzen, K. H. & Pahl, S. (1991). MODIFICATION OF DRY DEPOSITION IN A DEVELOPING SEA-BREEZE CIRCUATION-A NUMERICAL CASE STUDY. Atmospheric Environment., 26A(1), 51-61. https://doi.org/10.1016/0960-1686(92)90260-R.
Schlünzen, K. H., Bungert, U., Flagg, D. D., Fock, B. H., Gierisch, A. et al. (2012). Technical Documentation oft he Multiscale Model System M-SYS (METRAS, MITRAS, MECTM, MICTM, MESIM). MEMI Technical Report 3, Meteorologisches Institut, KlimaCampus, Universität Hamburg.
Schneider C., Pelzer, M., Tönges-Schuller, N., Nacken, M. & Niederau, A. (2016). ArcGIS basierte Lösung zur detaillierten, deutschlandweiten Verteilung (Gridding) nationaler Emissionsjahreswerte auf Basis des Inventars zur Emissionsberichterstattung, Umweltbundesamt UBA-FB-002360, Texte 71/2016, ISSN 1862-4804.
Schrödner, R., Tilgner, A., Wolke, R. & Herrmann, H. (2014). Modeling the multiphase processing of an urban and a rural air mass with COSMO–MUSCAT. Urban Clim., 10(4), 720-731. doi.org/10.1016/j.uclim.2014.02.001.
Schrödner, R., Wolke, R., Tilgner, A., van Pinxteren, D. & Herrmann, H. (2018). Modelling multiphase aerosol-cloud processing with the 3-D CTM COSMO-MUSCAT: Application for cloud events during HCCT-2010. Mensink, C., Kallos, G. (Ed.), In: Air pollution modeling and its application XXV: Proceedings of the 35th International Technical Meeting on Air Pollution Modelling and Its Application (Chania, Crete, Greece, 3-7 October 2016), Springer International Publishing, Cham, Switzerland, p. 587-592. doi:10.1007/978-3-319-57645-9.
Schubert, S., Grossman-Clarke, S. & Martilli, A. (2012). A Double-Canyon Radiation Scheme for Multi-Layer Urban Canopy Models. Bound.-Lay. Meteorol., 145, 439–468. doi:10.1007/s10546-012-9728-3.
Schultz, M. G., Stadtler, S., Schröder, S., Taraborrelli, D., Franco, B., et al. (2018). The chemistry–climate model ECHAM6.3-HAM2.3-MOZ1.0. Geosci. Model Dev., 11, 1695–1723. doi:10.5194/gmd-11-1695-2018, 2018.
Seinfeld, J. H. & Pandis, S. N. (2006). Atmospheric Chemistry and Physics: From Air Pollution to Climate Change. 2nd ed., John Wiley & Sons, New York.
Simpson, D., Benedictow, A., Berge, H., Bergström, R., Emberson, L. D., et al. (2012). The EMEP MSC-W chemical transport model – technical description. Atmos. Chem. Phys., 12, 7825–7865. doi.org/10.5194/acp-12-7825-2012.
Sofiev, M., Soares, J., Prank, M., deLeeuw, G., & Kukkonen, J. (2011). A regional to global model of emission and transport of sea salt particles in the atmosphere. J. Geophys. Res.: Atmos., 116. doi.org/10.1029/2010JD014713.
Solazzo, E., Bianconi, R., Vautard, R., Appel, K. W., Moran, M. D., et al. (2012a). Ensemble modelling of surface level ozone in Europe and North America in the context of AQMEI. Atmos. Environ., 53, pp. 60-74.
Solazzo, E., Bianconi, R., Pirovano, G., Matthias, V., Vautard, R., et al. (2012b). Operational model evaluation for particulate matter in Europe and North America in the context of AQMEII. Atmos. Environ., 53 (2012), pp. 75-92.
Steinbrecher, R., Smiatek, G., Köble, R., Seufert, G., Theloke, J., Hauff, K., et al. (2009). Intra- and inter-annual variability of VOC emissions from natural and semi-natural vegetation in Europe and neighbouring countries. Atmos. Environ.,43(7), 1380–1391. doi.org/10.1016/j.atmosenv.2008.09.072.
Stern, R., Builtjes, P., Schaap, M., Timmermans, R., Vautard, R., Hodzic, A., Memmesheimer, M., Feldmann, H., Renner, E., Wolke, R. & Kerschbaumer, A. (2008). A model intercomparison study focussing on episodes with elevated PM10 concentrations. Atmos. Environ., 42, 4567-4588.
Stockwell, W. R., Kirchner, F., Kuhn, M., & Seefeld, S. (1997). A new mechanism for regional atmospheric chemistry modeling. J. Geophys. Res.: Atmos., 102(D22), 25847–25879. doi.org/10.1029/97JD00849.
Stohl, A., Williams, E., Wotawa, G., & Kromp-Kolb, H. (1996). A European inventory of soil nitric oxide emissions and the effect of these emissions on the photochemical formation of ozone. Atmos. Environ., 30(22), 3741–3755. doi.org/10.1016/1352-2310(96)00104-5.
Tegen, I., Harrison, S. P., Kohfeld, K. E., Prentice, I. C., Coe, M. C. & Heimann, M. (2002). The impact of vegetation and preferential source areas on global dust aerosol: results from a model study. J. Geophys. Res., 107. doi:10.1029/2001JD000963.
Tõnisson, L., Voigtländer, J., Weger, M., Assmann, D., Käthner, R., Heinold, B. & Macke, A. (2021). Knowledge Transfer with Citizen Science: Luft-Leipzig Case Study. Sustainability, 13, 7855. doi.org/10.3390/su13147855.
Vignati, E., Wilson, J. & Stier, P. (2004). M7: An efficient size resolved aerosol microphysics module for large scale aerosol transport, J. Geophys. Res.: Atmos., 109. doi.org/10.1029/2003JD004485.
Weger, M., Heinold, B., Engler, C., Schumann, U., Seifert, A., Fößig, R., Voigt, C., Baars, H., Blahak, U., Borrmann, S., Hoose, C., Kaufmann, S., Krämer, M., Seifert, P., Senf, F., Schneider, J. &Tegen, I. (2018). The impact of mineral dust on cloud formation during the Saharan dust event in April 2014 over Europe. Atmos. Chem. Phys., 18, 17545–17572. doi.org/10.5194/acp-18-17545-2018.
Williams, E. J., Guenther, A., & Fehsenfeldi, F. C. (1992). An inventory of nitric oxide emissions from soils in the United States. J. Geophys. Res.: Atmos., 97(D7), 7511–7519. doi.org/10.1029/92JD00412.
Wolke, R., and Knoth, O., Implicit-explicit Runge-Kutta methods applied to atmospheric chemistry-transport modelling, Environ. Model. Softw., 15, 711–719, 2000.
Wolke, R., and Knoth, O., Time-integration of multiphase chemistry in size-resolved cloud models, Appl. Numer. Math., 42(1-3), 473–487, doi:10.1016/S0168-9274(01)00169-6, 2002.
Wolke, R., Schröder, W., Schrödner, R. and Renner, E. (2012). Influence of grid resolution and meteorological forcing on simulated European air quality: a sensitivity study with the modeling system COSMO–MUSCAT. Atmos. Environ., 53, 110–130. doi.org/10.1016/j.atmosenv.2012.02.085.